Saturday, November 3, 2007
Best Practices usage in Japan vs US Military Standards usage
In terms of reliability, the Japanese proactively develop good
design, using simulation and prototype qualification, that is
based on advanced materials and packaging technologies.
Instead of using military standards, most companies use
internal commercial best practices. Most reliability problems
are treated as materials or process problems. Reliability
prediction methods using models such as Mil-Hdbk-217 are not
used. Instead, Japanese firms focus on the "physics of
failure" by finding alternative materials or improved
processes to eliminate the source of the reliability problem.
The factories visited by the JTEC panel are well equipped to
address these types of problems.
Assessment methods. Japanese firms identify the areas that
need improvement for competitive reasons and target those
areas for improvement. They don't try to fix everything; they
are very specific. They continuously design products for
reduced size and cost and use new technologies only when
performance problems arise. As a result, most known
technologies have predictable reliability characteristics.
Infrastructure. The incorporation of suppliers and customers
early in the product development cycle has given Japanese
companies an advantage in rapid development of components and
in effective design of products. This is the Japanese approach
to concurrent engineering and is a standard approach used by
the companies the JTEC panel visited. The utilization of
software tools like design for assembly allows for rapid
design and is an integral part of the design team's
activities. At the time of the panel's visit, design for
disassembly was becoming a requirement for markets such as
Germany. Suppliers are expected to make required investments
to provide the needed components for new product designs.
Advanced factory automation is included in the design of new
factories.
Training. The Japanese view of training is best exemplified by
Nippondenso. The company runs its own two-year college to
train production workers. Managers tend to hold four-year
degrees from university engineering programs. Practical
training in areas such as equipment design takes place almost
entirely within the company. During the first six years of
employment, engineers each receive 100 hours per year of
formal technical training. In the sixth year, about 10% of the
engineers are selected for extended education and receive 200
hours per year of technical training. After ten years about 1%
are selected to become future executives and receive
additional education. By this time, employees have earned the
equivalent of a Ph.D. degree within the company. Management
and business training is also provided for technical managers.
In nonengineering fields, the fraction that become managers is
perhaps 10%.
Ibiden uses "one-minute" and safety training sessions in every
manufacturing sector. "One-minute" discussions are held by
section leaders and workers using visual aids that are
available in each section. The subjects are specific to facets
of the job like the correct way to use a tool or details about
a specific step in the process. The daily events are intended
to expose workers to additional knowledge and continuous
training. As a consequence, workers assure that production
criteria are met. Ibiden also employs a quality patrol that
finds and displays examples of poor quality on large bulletin
boards throughout the plant. Exhibits the panel saw included
anything from pictures of components or board lots sitting
around in corners, to damaged walls and floors, to ziplock
bags full of dust and dirt.
The factory. Japanese factories pay attention to running
equipment well, to continuous improvement, to cost reduction,
and to waste elimination. Total preventive maintenance (TPM)
is a methodology to ensure that equipment operates at its most
efficient level and that facilities are kept clean so as not
to contribute to reliability problems. In fact, the Japan
Management Association gives annual TPM awards with prestige
similar to the Deming Prize, and receipt of those awards is
considered a required step for companies that wish to attain
the Japan Quality Prize. No structured quality or reliability
techniques are used - just detailed studies of operations, and
automated, smooth-running, efficient production.
Becoming a black belt without Six Sigma
There are not so many articles who really explain Deming's methodology well because, though he is the founder of Quality with Walter A. Shewart, most of the "modern Quality Gurus" do not really understand his strange mixture between Management, Philosophy and Statistics. That's why I'm so pleased to be able to read this article:
Becoming a Black Belt without Six Sigma
by William H. Goodenow
Quality Assurance Manager
One of W. Edwards Deming's major contributions to the accumulated body of knowledge dealing with experimental design is his differentiation between enumerative and analytic studies. The key difference between the two is that the first deals with static (snapshot) conditions, while the latter deals with dynamic (changing) conditions. It is because of this fundamental distinction that the use of design and analysis tools which are intended for static conditions (traditional statistical methods) can be both inappropriate and ill advised for dynamic conditions.
There are other reasons for this concern. For example, most traditional methods of statistical analysis require that certain assumptions be met, the most common are:
- Normality of the data (a unimodal & symmetric normal--bell-shaped--distribution)
- Equivalence of the variances (equal variability in the test or response data)
- Constancy of the cause system (all non-controlled factors held constant)
These assumptions may or may not be met, or even tested for validity, before the analyses are performed--especially with the simplicity of using many of today's off-the-shelf statistical software packages.
In addition, Deming recognized that most symmetric (or composite) functions of a set of numbers almost always throw away a large portion of the actual information contained in the data. A traditional statistical test of significance is a symmetric function of the data. In contrast to this, a proper plot of data points will conserve the inherent information derived from both the comparison itself, and the manner in which the data were collected. For example, symmetric functions, such as the mean and standard deviation, can easily gloss over unknown and unplanned changes in the cause system over time, and, consequently, make a big and potentially misleading difference in the message the data are trying to convey for the purpose of providing a reliable prediction of future process behavior.
In other words, both the "design" approach and the methods of analysis typically taught and used for industrial experimentation leave much to be desired from a reliability of prediction point-of-view.
Design
Since the purpose of industrial experimentation is to improve a process and product's performance in the future, when some conditions may have changed, design efforts must provide for conducting the study over an appropriately wide range of conditions.
The actual determination of how wide to make this range is a critical part of the design stage of experimentation. If the range of conditions selected is too wide, the DOE team could falsely conclude that observed changes in the process will continue in the future when, in fact, these conditions may not be operationally feasible in the long run. If the range of conditions is too narrow, the team may miss important improvements that could result under a wider range of conditions. These errors are not quantifiable in analytic studies, and the reliability of any conclusions reached regarding future performance will be a function of how closely we follow Deming's design principles.
Other considerations include choosing the best variables for a study; handling background variables (those conditions to be either held constant or varied in an appropriately controlled manner), and nuisance variables; unknowns which can be neither held constant nor varied in a controlled manner; and deciding on replication, methods of randomization, the Design Matrix itself, planned methods of statistical analysis, cost and schedule.
There are still two considerations to be addressed:
What is the objective of the study? What background information do you already possess about the variables under study, in terms of their individual descriptive properties (mean, mode, median, standard deviation, the shape of their respective distributions, etc.), and the relationships between them.
Before establishing the objective, we must be as candid and complete as possible about the knowledge we already possess.
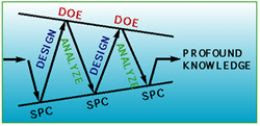
The problem with the "planners plan and doers do" mindset is the artificial separation it creates between the planning and execution steps of problem-solving. Deming recognized this tendency and much of his management and statistical thinking was directed at overcoming it. In Figure 1, we see how the idea of sequential learning not only applies to DOE, but how the process of stepwise experimentation, when properly used, can lead to the acquisition of profound knowledge.
To insure that the design process adequately provides for the execution of an appropriate and reliable experiment, it will be helpful to actually use some sort of design checklist and worksheet.
The reasons
A clue to the reasons why traditional analysis methods leave much to be desired in an analytic study was given in our discussion of the problems with symmetric functions alluded to by Deming and his followers--such as losing information inherent in the process and data collection activity. As troublesome as this tendency is, it does not represent the last straw. The fatal flaw shows up when future facts of life change sufficiently to render the original conclusions meaningless at best and clearly wrong at worst. It doesn't matter how big the F-Ratio is in the ANOVA Table, or other tests of statistical significance, they have no meaning if the conditions under which they were derived no longer exist in the same proportions as in the original study.
The alternatives
The best way to analyze data from an analytic study is to use the old stand-by methods of charting. This may include control charts of the SPC variety, variations of these or even the integration of SPC and DOE.
Figure 2 shows a slightly different way of diagramming the sequential application of the Scientific Method (Figure 1). Alternating uses of SPC and DOE can provide a concrete way of developing and exploring various process optimization hypotheses (DOE), and confirming the efficacy of these operational models in the future with carefully planned process control charts (SPC). As the difference between our theories and the facts narrows, knowledge grows.
The really important aspects of control chart analysis are that it: takes into account the order in which the data were generated and collected; and does not require assumptions of normality, equivalence of the variances or constancy of cause systems, since the data in the experiment allows us to reject any or all of these hypotheses. It allows us to study the individual data points, groups of data points, and various patterns over time that may provide valuable clues as to why certain measurement points showed different results than others.
It is this last benefit that forms a fundamental and extremely powerful basis for the analysis of any analytic study. Not only would we have been misled by traditional statistics, but a cursory examination of the control charts, as well, could have allowed us to not really see what the data were trying to tell us.
About the author:
William H. Goodenow has been an examiner with the Wisconsin Forward Award (WFA) program, Wisconsin's adaptation of the Malcom Baldrige Quality Award.